Tech Development Research
An academic study to understand users and utility of an AI tool in development.
THE CHALLENGE
An AI dog behavior data pipeline is in development. To test this tool's utility and benefits, we need to know what people see and think when looking at dogs, too.
To solve this, I conducted a live dog interaction experiment and used select clips in a survey to understand what UK adults see and believe about dog body language and excitability, given their background with dogs.
​
I designed the study to create a standardized and comparable test applicable to the tool's use-case. The design was carefully constructed to encourage balanced groups, reduce bias, and mitigate effects of presentation order. Participants saw block-randomized clips of dogs being either rewarded or ignored as our means of controlling for dog emotion (affect in a more technical term). Bayesian analysis was used to estimate predicted responses so the insights were most useful for our needs.
With careful planning and selection, I revealed insights that altered the development of the tool and indicated future directions for use.
Context
Academic research related to tool development
Role
Lead researcher
Timeline
About 6 months, part-time
Aims
-
To see whether people recognize differences in dog body language, excitability and emotion across more and less enjoyable interactions, taking into account aspects of the person's background and the dog they're evaluating.
-
Review what body parts and movement people report to be looking at when assessing dog emotion.
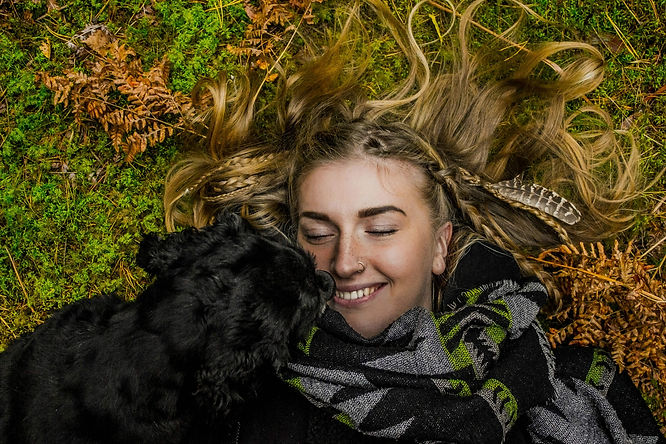
Methodology
-
434 participants from aim of 450 based on target UK population (later the population was expanded so this is no longer reflective of population views).
-
Quantitative data from binary questions and 5-point Likert scale rating.
-
Qualitative data from explanation text box.
​
The survey asked general questions:
-
If they own/owned a dog.
-
If they were a professional or volunteer working with dogs (vet, trainer, shelter worker, etc).
-
If they took a class on dog body language.
For each dog video, we asked:
-
Do you know this dog?
-
How much do you think this dog is expressing positive or negative emotion?
-
Is there anything you observe about the dog that influenced your answer?
-
What emotions would you guess that this dog is feeling?
-
How excited or energetic would you guess this dog is being?
Layout of the Survey
Participants were shown one clip per page. Each page repeated the instructions and all questions had an information icon that showed an elaboration and an example.

Analysis
-
I coded a blinding script and another researcher ran it to get the blinded data.
-
Data cleaning and analysis in Python, except Bayesian statistics done in R.
-
Used Spearman's correlations to look between demographics and responses and cross-tabulation to look at proportions of people who were trained in dog behavior and those who work with dogs.
-
Text and sentiment analysis using NLTK and VADER to look at qualitative data.
-
Bayesian ordinal logistic regression models were used to see factors that influenced scoring of dog emotion and for looking at dog excitability.
-
The dog's Likert score was compared to the grand mean of all dog scores.
-
Cumulative logit model was used as the distances between Likert scale responses was unknown and non-normal distribution.
-
For both models, samples were drawn from NUTS, an extension of MCMC algorithm, with 3000 iterations, 1000 iteration warmup, and adapt delta of 0.95.
-
The formula looked like Emotion ~ Treatment + Dog_Name + OwnDog + (1|Response_ID)
-
​​​
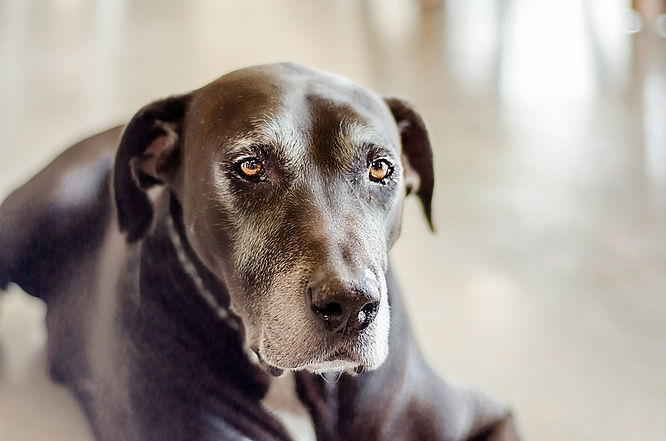
Results lead to new questions
Individual differences were stronger predictors of people's ratings than treatment group. Maybe age played a part in this? Breed? Temperament?
We'll need another study to explore this!
Discussion
NOTE: results are sensitive given publication status and so are not included in this case study.
-
The survey was very effective in identifying the most frequent body parts. I was able to compare the model outcome with human ratings of the same data, the most important purpose for this project.
-
​Diversity of expression between individuals was more important than the treatment group. This has implications for the data pipeline and might mean we consider pivoting to a more useful project.
-
The qualitative data complemented the quantitative results and supported that people describe dogs differently by group, with + language for dogs in the reward group.
-
Results suggested differences between scale responses and free text. This implies that having a text entry response and a rating response cannot be used interchangeably. This is important when designing future surveys on this topic.